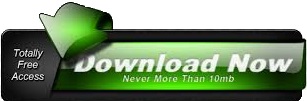
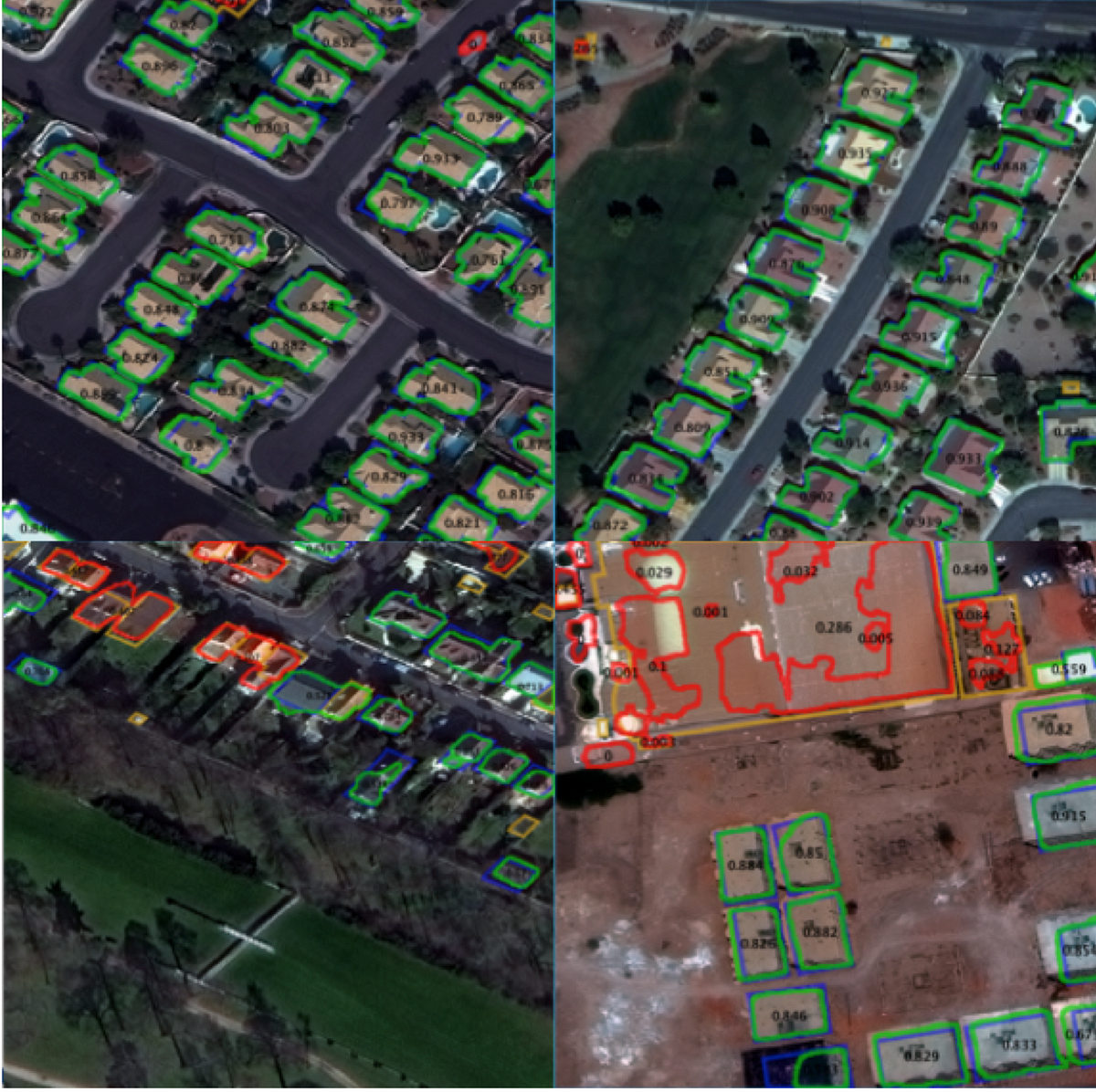

The results demonstrated that the proposed framework using keypoint estimation exhibited better segmentation performance when compared with Mask R-CNN in terms of both qualitative and quantitative results.īuildings provide key cadastral information related to populations and cities, and are fundamental to urban planning disaster management and 3D city modeling. Our model achieved an F1-score of 0.650 with an mIoU of 62.6 for building footprint extraction using the OpenCitesAI dataset. A building polygon is created by grouping the predicted keypoints through a simple geometric method.
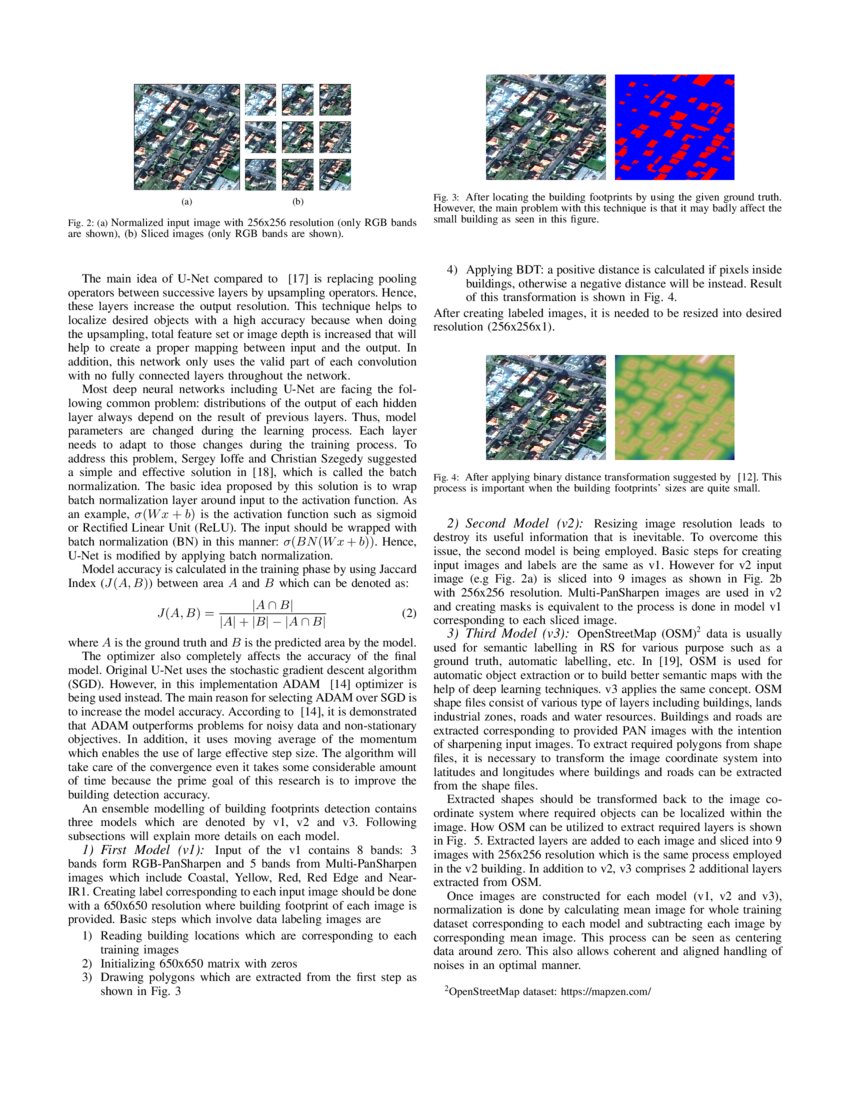
Keypoints between instances are distinguished by merging the rough segmentation masks and the local features of regions of interest. The proposed framework follows a two-stage, top-down approach that is divided into object detection and keypoint estimation. The target keypoints in building extraction are defined as points of interest based on the local image gradient direction, that is, the vertices of a building polygon. The objective of this study is to generate visually enhanced building objects by directly extracting the vertices of individual buildings by combining instance segmentation and keypoint detection. However, semantic segmentation produces coarse results in the output, such as blurred and rounded boundaries, which are caused by the use of convolutional layers with large receptive fields and pooling layers. Deep convolutional neural networks successfully perform footprint extraction from optical satellite images. Building footprint extraction is an active topic in the domain of remote sensing, since buildings are a fundamental unit of urban areas.
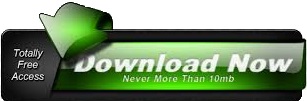